William Jarrard
I am currently a Data Scientist and Machine Learning Researcher at UCSF where I work under Felix Feng on applying deep learning to study transcription factors in prostate cancer. I was previously an undergraduate at UVA, where I majored in Systems Engineering. During my time at UVA, I made significant contributions to cancer research, particularly in advancing our understanding of genetic factors in oncology and helping to develop a method for extracting crucial information, like drug names, from paper medical sheets for a hospital in Rwanda. I also completed a 4+1 program to earn my Master's in Data Science at UVA.
Get in touch →
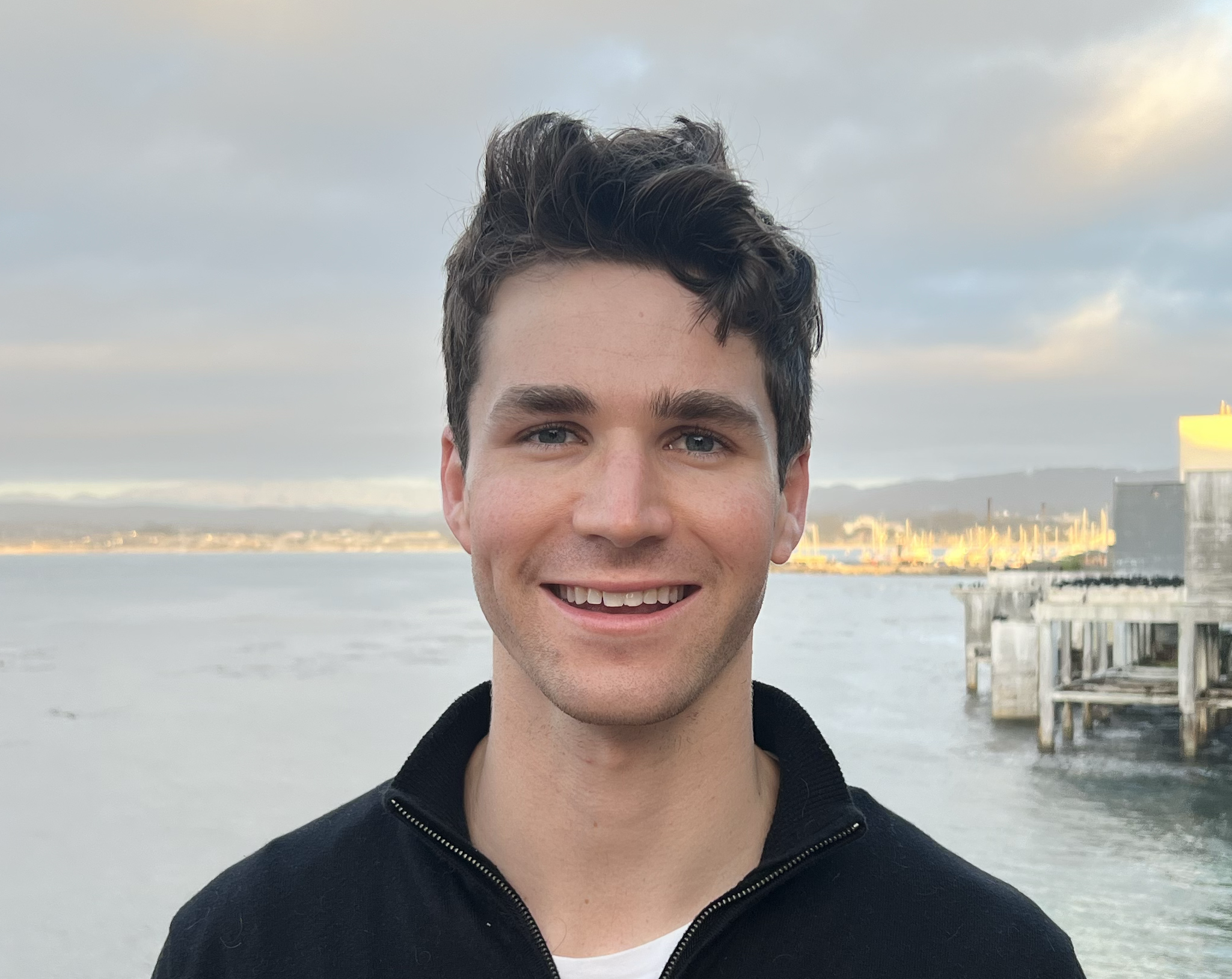
Work Highlights
Studied how UTX, a histone demethylase and tumor suppressor, relies on phase separation into liquid condensates for its chromatin-regulatory activity. Loss of its intrinsically disordered region (cIDR), common in cancer mutations, disrupts this process, impairing tumor suppression and chromatin interactions.
Go to →
Developed a method for extracting crucial information, like drug names, from paper medical sheets. By utilizing YOLO for segmentation and LSTMs to capture the sequential nature of drugs, out method achieved impressive accuracy rates of 95% and 90% for drug name and drug dosage extraction, respectively. This project has the potential to streamline the medical record-keeping process and improve patient care.
Go to →
Investigating transcription factor colocalization in prostate cancer using an innovative deep learning model to understand the neuroendocrine and adenocarcinoma phenotypes.
Go to →
Personal Projects
Gold-Adjusted SPY Index
A visualization understanding when trust in money breaks, assets priced in that money often rise not because they gained value, but because the money lost it.
View project
rust-seq
Helping out with the development of fundamental building block crates for rust in bioinformatics.
View project
UVa Building Classifier
Classified buildings at UVa using transfer learning.
View project
Comparing different ML Methods for Song Classification
This project focuses on classifying songs into genres using only their lyrics, evaluating the effectiveness of both traditional machine learning algorithms and neural networks in accomplishing this task.
View project